CFA Institute 2024
Using NLP to Unlock a Treasure Trove of Alternative Data
Overview
Investment professionals have long looked to alternative data sources to generate alpha. Advances in artificial intelligence and natural language processing have made such insights far more accessible.
Financial data often provides a poor approximation of a company’s current health in a fast-moving world. The release of quarterly results can lag a shift in earnings trajectory that took place more than three months previously. This can be consequential if, say, in the intervening period, a brand had released a blockbuster product or been caught up in a scandal that alienates its customer base.
In a bid to overcome this gap, investors have looked beyond financial data for an informational advantage since long before the era of big data – for example, by cultivating contacts within specific industries and officialdom or doing legwork to gauge retail footfall and consumer reactions to new products. But now, amid an explosion in the volume of available data and advances in data analysis, alternative data can be used in a far more systematic way.
Deloitte recently highlighted the bright prospects of alternative-data providers, who offer access to data sets including, among many others, credit card transactions, geolocation records, energy meters, motion sensors, social media posts, search engine queries, news feeds, satellite images and weather information.
These can provide a variety of valuable signals. “If you’re a real estate investor, for example, you could use heat or energy indicators to look at the occupancy of buildings, or look at highway traffic to determine whether people are commuting into offices,” explained Sri Krishnamurthy, CEO and Founder of QuantUniversity.
Given that potential, Deloitte expects the combined revenue of alternative data providers to overtake that of traditional data vendors, ballooning to USD137 billion by 2030 (see Figure 1). Demand will be fueled in large part by the investment industry.

The more widely available alternative data sources become, however, the more difficult it will be to achieve alpha using them. And given that the window between such data remaining alternative and going mainstream is likely to shrink, investment managers need to move quickly to leverage its full potential before its value is eroded. Those that fail to do so could be operating with an informational disadvantage, putting them at risk of falling behind their competitors.
Making Sense of Unstructured Data
Of course, using alternative data is complicated by the fact that much of it – at least initially – is unstructured, not digitized and therefore not readily processable. Much of this unstructured data, which accounts for 80-90% of all new data, comes in text, image or voice formats.
“Unstructured data reflects a lot of business value, which is currently not exploited by data science because we focus on structured, numerical data,” said Isaac Wong, an Assistant Fund Manager at eFusion Capital.
But that is changing quickly (see Figure 2). In particular, text data, ranging from earnings call transcripts and company filings to social media and blog posts, is being increasingly leveraged to enhance investment insights and build better portfolios. This has been made possible by big strides in natural language processing (NLP) techniques, which enable machines to interpret and analyze human language.
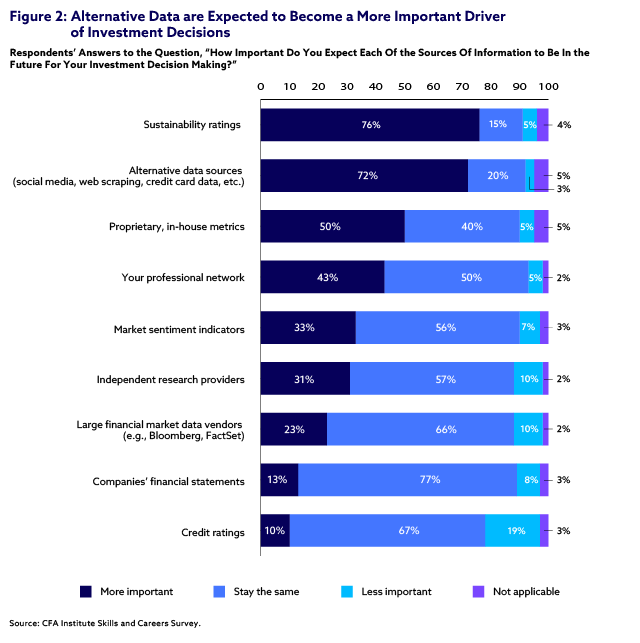
NLP has been used by investment professionals to summarize and extract key messages from tens of thousands of pages of earnings call transcripts, securities filings and corporate actions – resulting in alpha generation not explained by traditional risk and return factors.
NLP can also be used to extract context, meaning and intent from written or spoken content. Through a technique known as sentiment analysis, algorithms are applied to news articles, social media, and other data sources to gauge how people feel about specific products and topics.
This can help with both explaining and predicting shifts in stock prices. “Investors can look at social media to look for significant trends when people are discussing certain products, or if Elon Musk makes a statement, to see whether there is movement,” said Krishnamurthy.
One of the earliest use cases for sentiment analysis in the investment process was summarizing customer reviews and comments on social media and other platforms. This can be demonstrated using the example of Starbucks’ launch of a new rewards program in February 2016, which led to many customers taking to what was then called Twitter to protest the changes.
As shown in Figure 3, there was a marked uptick in “angry” and “dislike” tweets in relation to positive and neutral ones following the initial announcement up to the rollout of changes a month later. This insight could have allowed investors to anticipate the potential financial impact of the rewards program changes ahead of the official quarterly results announcement in April.

Sentiment analysis has become much more sophisticated in the intervening period, with the transformer-based approach used by large language models (LMS) such as ChatGPT able to factor in the nuances of sentence structure and semantics on the meaning of text. This was demonstrated recently in ChatGPT’s ability to decipher “Fed Speak” in the following statement: “Labor market conditions have shown some improvement in recent months, on balance, but the unemployment rate remains elevated.”
Not only could ChatGPT classify the statement as dovish, but it also explained its reasoning (see Figure 4):
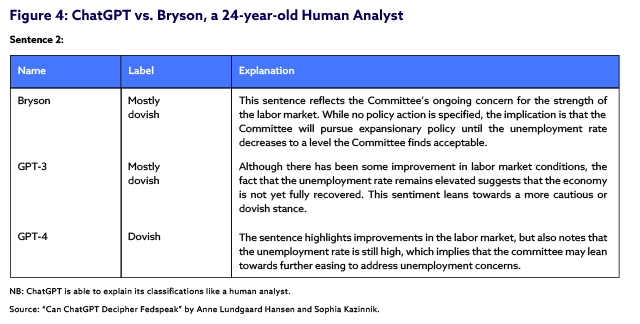
LLMs like ChatGPT are remarkably powerful, versatile and accessible, giving them potential to serve as an equalizer for big and small organisations in exploiting a broad array of unstructured data, according to Wong.
“For instance, you could give ChatGPT a PowerPoint from a company’s annual investor conference presentation, explaining its business plans over the next decade. ChatGPT — with some additional plug-ins — can analyze the content, summarize it, and compare it to previous PowerPoint presentations,” said Wong.
The Limits of NLP
But NLP is not quite a silver bullet for dealing with the reams of unstructured data that are yet to be exploited by investment professionals.
For one thing, sentiment analysis could lead to faulty assumptions and predictions because what people say often does not correspond with what they do. They could, for example, lambast an advertisement for a new product and still ending up buying it.
Another issue is that alternative and unstructured data are often only available for a limited universe of stocks, making them difficult to apply systematically.
And the technology itself has important limitations. Despite the advances made in decoding nuance, human language – which depends on complex grammatical rules, idiomatic usage and contextual understanding – remains difficult for machines to process, interpret and act on.
This is in part a result of the need to convert text into numbers suitable for digital computation, which tends to simplify the intricate structure of language by omitting or weakening critical relationships among words, phrases, and sentences. That could make subsequent analysis of text data prone to error.
The domain-specific uses of language in finance also present a challenge. The meaning of words like ‘yield’ or ‘hedge’ may seem obvious in a financial context, but programs need to account for the distinct semantics of technical terms and specialized vocabulary. This has prevented the transfer of useful applications from other domains, such as online search and marketing, to the financial realm.
These limitations could soon be overcome as the technology continues to advance, making it possible to leverage NLP and alternative data even more widely in the investment process.
While experts do not believe that alternative data can entirely replace financial data in the investment decision process, it will increasingly provide a valuable complement, highlighting angles that may have been missed by traditional methods.
In a world awash in alternative data, the difficulty will lie in determining what is worth focusing on, and judging relevance and reliability. This will be facilitated by clear and unfettered communication between data scientists and investment professionals with subject-matter expertise.
Through a collaborative effort, the investment industry can more fully leverage the power of alternative data and NLP to paint a clearer, quicker picture of the world, enhancing opportunities to generate alpha.
Note: With thanks to Ingrid Tierens, Head of Data Strategy for Global Investment Research at Goldman Sachs, for sharing insights conveyed in this article.
View more Data & Technology stories